What is prescriptive analytics?
Can Prescriptive Analytics help your organization? And what are the differences between predictive and prescriptive analytics?
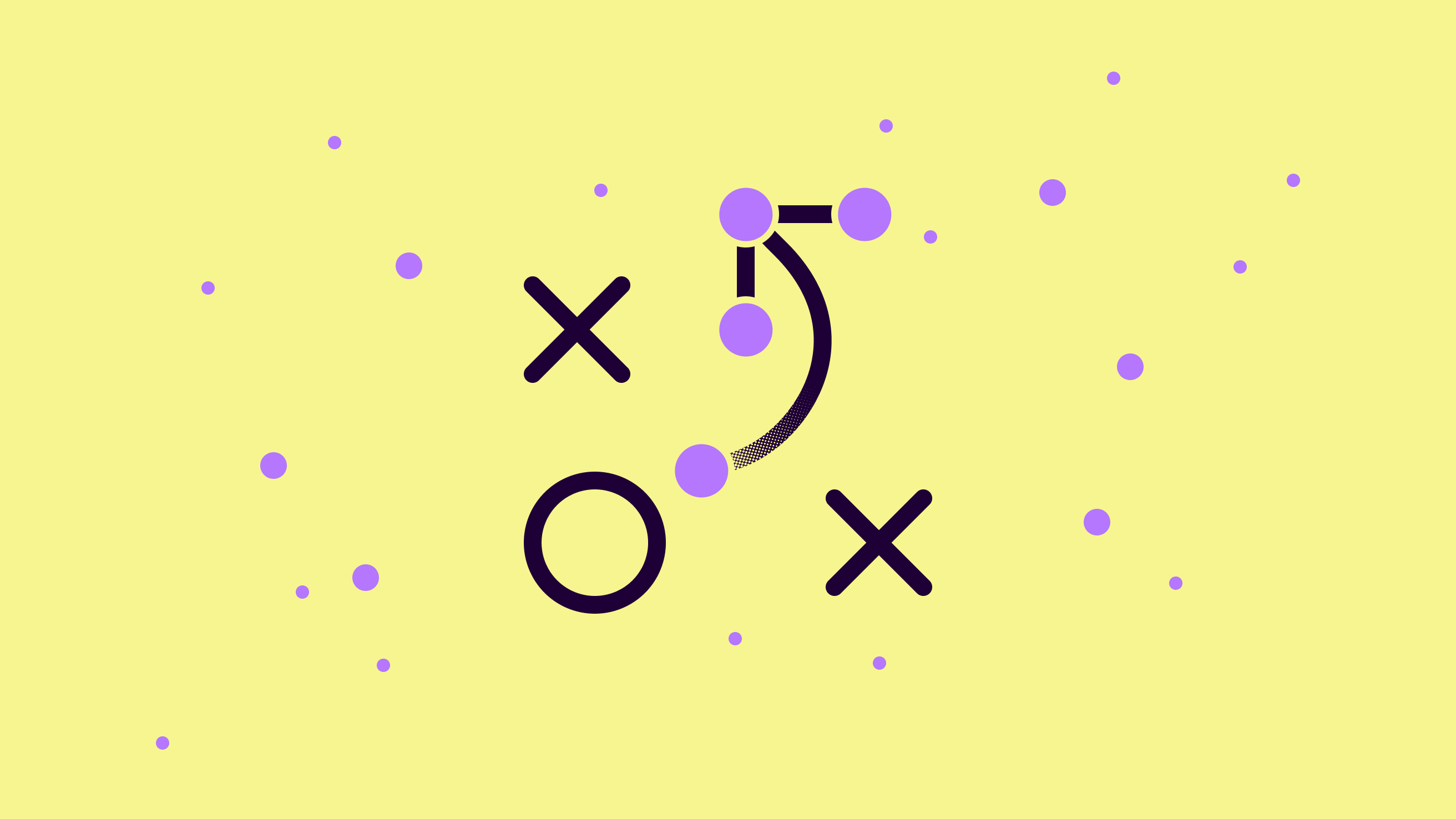
Discover:
- Prescriptive analytics definition
- What’s the difference between predictive analytics and prescriptive analytics?
- How does prescriptive analytics work?
- Examples of prescriptive analytics in online learning
- Descriptive vs predictive vs prescriptive analytics
Learning Analytics is not simply about collecting data from learners, but about finding meaning in the data in order to improve future learning.
To do this, learning analytics relies on a number of analytical methods: descriptive analytics, diagnostic analytics, predictive analytics, and prescriptive analytics.
Prescriptive analytics definition
Prescriptive analytics is a statistical method used to generate recommendations and make decisions based on the computational findings of algorithmic models.
For learning analytics, this could range from simple automated recommendations made to employees who are taking online training, to recommendations that indicate how instructors or course designers can improve the design of a course or program.
At present, prescriptive analytics is not widely used in the field of learning & development due to the complex requirements needed in the field of machine learning. It can be found in adaptive learning and also within some learning experience platforms (LXP).
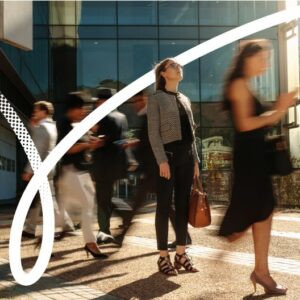
Use learning data to accelerate change
Understand learning data and receive a practical tool to help apply this knowledge in your company.
Download workbookWhat’s the difference between predictive analytics and prescriptive analytics?
Prescriptive analytics is considered an extension of predictive analytics.
An insightful forecast from predictive analysis can be analyzed using specific models designed for prescriptive analysis in order to produce automated recommendations or solutions. Prescriptive analytics require complex algorithms in order to accomplish such machine-based decision-making.
With predictive analytics, it is understood that predictions may or may not happen. For prescriptive analytics, however, there is an element of risk when using automated recommendations: human behavior can be unpredictable. Statistical models that base an outcome on the analysis of human behavior requires a degree of caution.
How does prescriptive analytics work?
Generating automated decisions or recommendations requires specific and unique algorithmic models and clear direction from those utilizing the analytical technique. A recommendation cannot be generated without knowing what to look for or what problem is desired to be solved. In this way, prescriptive analytics begins with a problem.
Here’s an example:
A Training Manager uses predictive analysis to discover that most learners without a particular skill will not complete the newly launched course. What could be done? Now prescriptive analytics can be of assistance on the matter and help determine options for action. Perhaps an algorithm can detect the learners who require that new course, but lack that particular skill, and send an automated recommendation that they take an additional training resource to acquire the missing skill.
The accuracy of a generated decision or recommendation, however, is only as good as the quality of data and the algorithmic models developed. What may work for one company’s training needs may not make sense when put into practice in another company’s training department. Models are generally recommended to be tailored for each unique situation and need.
Data governance strategy
Prescriptive analytics also warrants a degree of caution from the standpoint of ethics.
Generating automated decisions or recommendations based on a computer’s interpretation of student data can create some questions surrounding privacy and fairness. Did learners give consent? Who has access to the data and results?
If the data collected is not an entirely accurate picture, then the predictions made about the learner could be inaccurate, which could then lead to incorrect recommendations or decisions being made for or about the learner.
It is recommended that a data governance strategy be put into place and that an emphasis on validation for the prescriptive models be taken.
Examples of prescriptive analytics in online learning
The use of prescriptive analytics is growing and can already be found in some popular learning management systems (LMS) and learning technologies:
- There are some tools that use prescriptive analytics to identify what content the learner has already learned so that new content not yet mastered is presented instead. This is an example of how prescriptive analytics is finding its way into adaptive learning.
- Some LMS’s enable administrators to define specific rules in order for automated feedback or actions to take place; for example, if an employee is struggling to complete a training course, the system may recommend they look at a different resource to obtain skills needed for the previous course.
- Some LMS’s are promising to reduce training time for employees by determining previous knowledge and proficiency baselines in order to recommend which training courses or resources are best suited for the learner.
Although the use of prescriptive analytics seems fairly small scale for the moment, it is sure to evolve steadily over the years as machine learning and Artificial Intelligence (AI) becomes more accessible.
Descriptive vs predictive vs prescriptive analytics
Descriptive Analytics is focused solely on historical data.
You can think of Predictive Analytics as then using this historical data to develop statistical models that will then forecast about future possibilities.
Prescriptive Analytics takes Predictive Analytics a step further and takes the possible forecasted outcomes and predicts consequences for these outcomes.
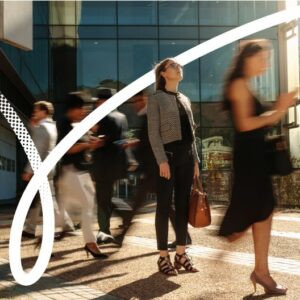
Use learning data to accelerate change
Understand learning data and receive a practical tool to help apply this knowledge in your company.
Download workbook