Personalized learning
Here we take an in-depth look at personalized learning and how to use it to best effect in your employee training programs.
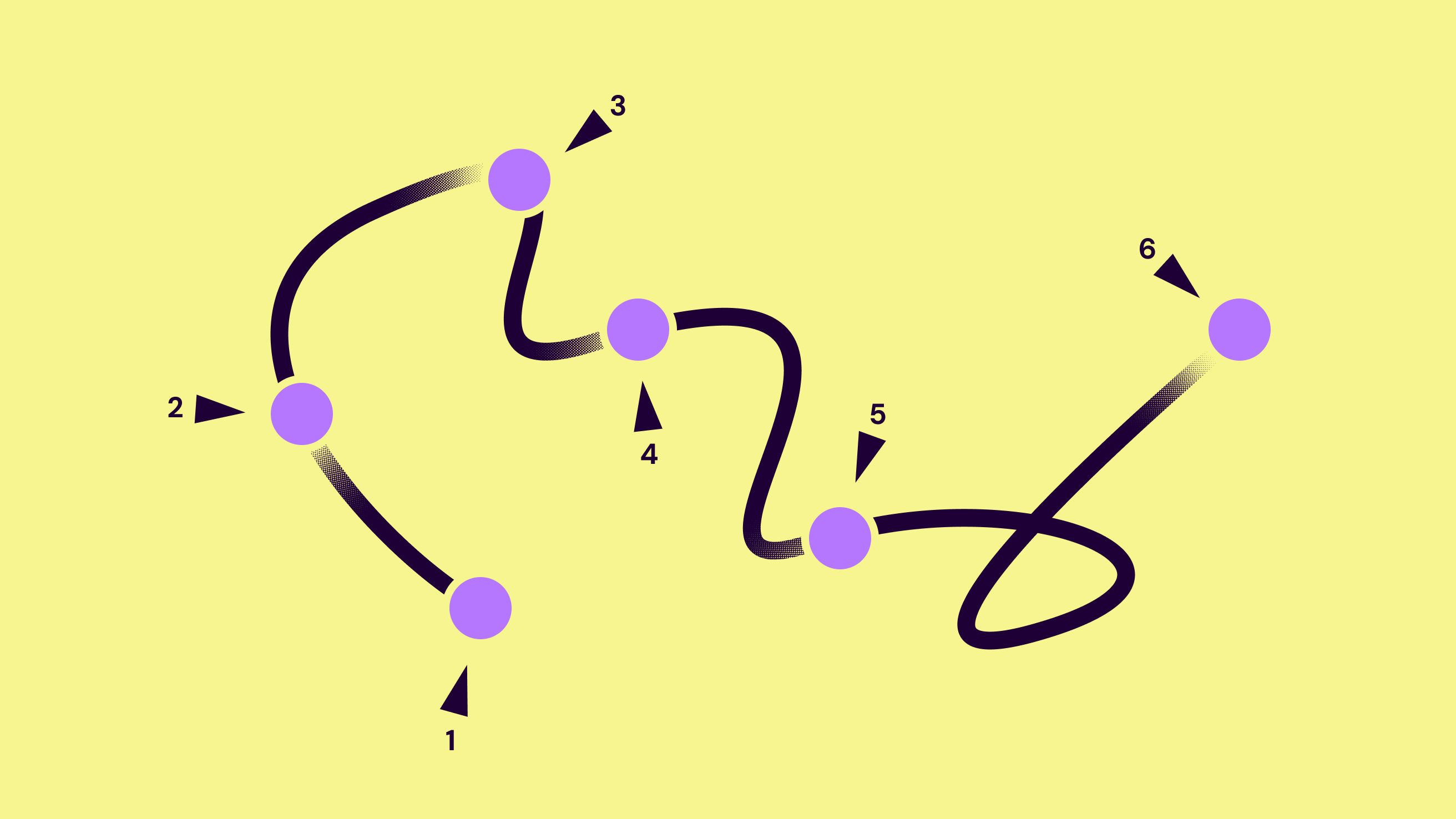
This guide will give you an in-depth look at personalized learning, what it is, and how you can effectively implement it within your organization.
After reading this guide, you will be able to achieve better results in your employee training programs by taking advantage of personalized learning.
Discover:
- What is personalized learning?
- How does personalized learning work?
- Why is personalized learning important?
- Benefits of personalized learning
- Examples of personalized learning
- A new era of personalized learning with AI
- How to get started with personalized learning in organizations
- How can learning be made even more personalized and effective?
- How can modern technologies help with personalized learning?
- How do you make a personalized learning plan?
- Conclusion
What is personalized learning?
Personalized learning is the customization and adaptation of educational methods and techniques so that the learning process is better suited for each individual learner, with their own unique learning style, background, needs, and previous experiences.
Learning can happen in a myriad of different places, activities, methods, and time frames.
From the lecture hall populated with hundreds of learners listening to an instructor to a one-on-one mentorship program, from interactive online games to complex technical textbooks, there are so many formats that learning can take.
There are many different learning techniques, as well as many styles of teaching and learning, all of which combine to make every learning experience different and personalized. Each type of learning has pros and cons and will offer different things to different learners.
In a personalized learning approach, the learner’s personal experience, knowledge, and habits are connected with learning methods, so that they can learn faster, understand new concepts more easily, and improve their learning performance.
How does personalized learning work?
Personalized learning centers around the task of connecting a learner’s previous knowledge, experiences, and abilities with training materials that will link that understanding with new information.
The simplest example of personalized learning would be when an instructor provides learning material with proper content and context, and in the best way for the learner.
This is done by using the existing knowledge that the instructor has of the student. The instructor understands how best to connect the learner’s previous experiences and abilities to the new information, building links between existing knowledge and new information.
Proper learning material is content that is relevant to the learner’s previous experiences.
The best way, for each learner, is delivery of information in such a way that the learner is able to acquire the new information easily.
This could be the type of material (video, text, or interactive games, for example), the time spent, the amount of material covered in each session, and the order in which new information is explained. This will vary for each learner, as everyone has different learning styles.
This, of course, is not a scalable solution, but it is the simplest explanation of how personalized learning can be actioned. It is a process of connection, of delivering the right tools to assist the learning path.
Nowadays, to make this type of approach happen, and more importantly, be scalable, organizations need to be able to create a digital learning infrastructure that can automate this process and make it cost-effective.
To do so, organizations need to use a variety of digital solutions. These can include communication channels (e.g. Slack), AI technologies (e.g. machine learning and automation), data analysis, learning platforms, mobile technologies, and more.
Only by creating a system using the many available tools can an organization better understand the needs of each individual learner, and deliver the content that they need, when they need it, in a truly scalable and organized fashion.
Let’s take a look at how this would work in practice. Meet Jeff, your new employee.
You know the following information about Jeff:
- He has an engineering degree.
- His previous work experience was as a senior engineer at a construction company.
- He prefers to consume video content rather than read.
- He enjoys using technology, and quite often uses mobile devices in his work.
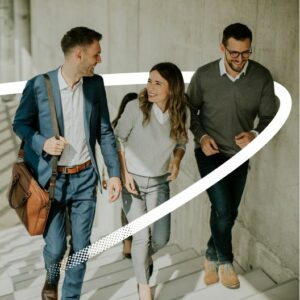
Build a learning culture: a practical workbook for your organization
Upgrade your organization’s learning culture with clear, actionable strategies to address the challenges.
Download workbookSome learner profiles will have more variables, but let’s start with this.
So based on those parameters, a learning instructor would create a specific learning path for Jeff. The path that will be based on his education (comfortable with high-level educational content), experience (connecting information to what a senior engineer would know), and preferred content type (video) and will deliver it on the go, so Jeff can engage with content on mobile devices.
So this is how personalized learning looks like and works.
Why is personalized learning important?
If an organization wants to improve learning outcomes, personalized learning is a proven way to do so.
By taking data related to a learner’s previous experience and linking that to new concepts, personalized learning results in a more complete understanding of new concepts, better engagement, and knowledge retention is improved. In short, personalized learning makes learning more effective.
By looking at the previous example, you can see how personalized learning can transform a common training program and make it more personalized for each unique user. Such changes make the learning process engaging, faster, and more effective.
Even making learning content slightly personalized will make a difference, for example, removing material that is unnecessary while highlighting the training that will help the learner most.
As technology advances, learners become more demanding, and they expect training programs to keep up. Nowadays, personalized content is everywhere. We have personalized feeds on social media, personal playlists, movie recommendations, and a lot of other things. We already expect that any new tool will have something similar. At work, we expect that the learning platform will offer us something new, relevant exactly for us.
Without updating the way that learning is delivered, organizations run the risk of disengaging employees in a vital area.
Not only that, consider what a new employee will think if they begin their onboarding process with a company that uses an outdated, non-personalized approach to training. Will they trust that this organization can help them upskill throughout their career? Will they take the organization seriously as a competitive and forward-thinking company to work for?
Employees consistently report that they look for jobs that will offer training and opportunities for growth, and will stay longer in roles that provide them. As employees learn, they are able to improve their performance, delivering better business results.
Good employee training programs can increase revenue, boost productivity, and breed innovation within an organization. As more organizations face the challenge of upskilling workers effectively and efficiently, personalized learning has come into the spotlight as a method that can deliver that in a scalable manner.
Benefits of personalized learning
1. Saves time
Personalized learning cuts down on the time it takes a learner to engage with and understand a new subject.
It also serves to remove content that is no longer relevant or would be redundant due to the experience level of the learner, saving time that would otherwise be wasted on learning concepts that will not serve the learner.
2. Increases engagement
When content is both relevant and personalized, learning is more engaging.
A learner is more likely to interact with, and remember content that targets their current role, projects, or area of work.
3. Improves knowledge retention
When content is based on previous experience, the learner will retain that information for a much longer period of time.
When a personalized learning path connects each piece of the puzzle together, with each piece supporting each other to intertwine the information, the learner will be able to better recall information by linking it to existing knowledge.
4. Increased motivation
Learning that is connected to something relevant, be it the learner, their job, or their hobbies will increase motivation for the learner.
This is especially true if the content contains tips or helpful information that is immediately actionable.
5. Improves learning results
Studies have shown that a personalized learning approach yields better learning results. This approach elevates learning and provides content that is relevant, engaging, actionable, and memorable.
The end result is a learner who is pleased with their interaction with the material and is better at their job.
Examples of personalized learning
1. One-on-one Tutoring
In a situation where you have two individuals, a learner, and a teacher, you need the teacher to understand exactly what the learner already knows, and then tie that to new information.
Consider this example of a parent teaching a child about cars: Recently my son asked me “what is this and what do you need it for?” about the gearshift in my manual transmission car.
After thinking about it a moment, I explained it to him by talking about his bike’s shifter and gear mechanisms, which allow him to change the speed/effort ratio.
I then connected the concept of those mechanisms to the gearshift in my car. By knowing something from my son’s previous experience and linking new information to that, I made this new concept easy for him to learn.
What if the parent chose to explain this concept using diagrams of engines and gearboxes, talking in high-level mechanical engineering language?
The kid would have checked out immediately, as he would have been unable to connect this new information to what he already knew. No learning would happen, and the child likely wouldn’t ask any more questions like this to his parent, as he has learned that the answer will be incomprehensible.
This is how one-on-one personalized learning can be achieved by creatively using what is already known about the learner, and linking that to new information.
2. Mentoring
One of the well-known ways of personalized learning is mentoring. The more experienced employee is assigned as an adviser to the less experienced employee.
With all knowledge that the mentor gathered by experiencing something in the past, he could understand the issues and difficulties that the mentee is facing and guide the learning towards understanding.
This model works great, but its limitation is in its scalability.
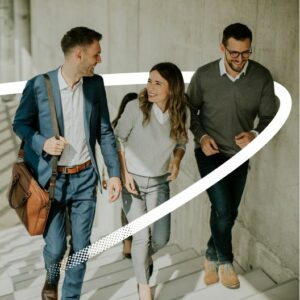
Build a learning culture: a practical workbook for your organization
Upgrade your organization’s learning culture with clear, actionable strategies to address the challenges.
Download workbook3. Online course providers
You have definitely seen these type of courses, and very probably used one yourself.
Coursera, edX, Linkedin Learning, OpenSesame, and many, many others. In all of those services, you have a personal account that the system tracks and stores all of your learning data in order to offer you new relevant courses.
When you finish one course, the platform will offer you advanced courses or materials relevant to the subject.
4. Search engines
Search engines are the most popular way of personalized learning. Google answers your questions with personalized answers.
If you like to read, you might order books online, or visit some publication sites to find a new book, Google will remember that. So if you google “Harry Potter”, the system will offer you the best offers from online book shops. At the same time, if you like to watch movies, and do it quite often online, you will see movie-related answers.
Even the subject is the same, “Harry Potter”, Google knows that the subject contains a different type of information.
Knowledge Graph, the technology behind that, connects different aspects of the subjects together.
So, in the end, you can see the results that are most relevant to you. Harry Potter is a book, movie, character, and you even will see pictures and profiles of Daniel Radcliffe, the actor who played the character.
All of this happened because Google wants to provide the most relevant information to the users as possible, and uses personalized learning to do so.
5. Corporate training example
Air Methods, a Colorado-based helicopter medical transport company used personalized learning to boost their pilot training program.
Using a cloud-based learning system, they turned to artificial intelligence to pinpoint the topics in which pilots were struggling and present more information and differently worded questions to ensure that pilots truly knew the topic.
By using frequent, short quizzes and games, the pilots were engaged and the organization was easily able to pinpoint areas where more training was needed.
This use of personalized learning allowed the company to cut in-person, instructor-led training sessions in half, and also reduced the number of days needed for onboarding, from ten to five days.
A new era of personalized learning with AI
As artificial intelligence technologies become more advanced, more able to distinguish individual needs, and more customizable, their use in personalized learning has increased.
The two biggest components of a successful personalized learning program are data and AI, specifically machine learning.
By using data, an organization can teach AI to recognize patterns, make connections between information and knowledge, and provide the correct information at the right time to the user.
The biggest hurdle in this is collecting and analyzing data about employees.
How to get started with personalized learning in organizations
So, what should an organization do if they want to implement personalized learning?
How can a business help an employee to not only find out the information they need but to also ensure the answer is linked to their previous experience?
The answer is a combination of sophisticated search and personalization engines.
First, a search engine will narrow the possible sources of information, like documents, web pages, or training materials, and then a personalization engine will prioritize the content that is most relevant to the employee’s previous experience.
The search engine is something that we are accustomed to using every day, like Google.
In a corporate environment, it could be a separate engine for each information system or some sort of more advanced engine that is able to query many different backend systems and combine results.
But how can an organization make those results more personalized, e.g. related to the employee’s previous experience?
Or to be even more precise, how do we know what is the employee’s previous experience?
How can an employee’s experience be digitalized?
1) The first step in that direction is to list for each employee what skills they have learned previously and what is their level of knowledge.
It could be done, for example, by analyzing their CV using text analytics or by asking them to fill in a simple form with skills in rows and knowledge levels in columns (skills matrix).
This information already gives some clues, but not with much detail, though. In addition, this is a pretty much static view, which doesn’t take into account the learning and experiences happening after those skills had been listed.
2) In the second step, in addition to the Competence Matrix, collected in the previous step, HR needs to collect information about training attended, certifications achieved, and everything else related to formal learning.
For the employee, this could improve the relevance of the information found significantly, as the level of their knowledge is known to the system and is updated in accordance with formal learning attended. As a result, for example, expert-level information will not be shown to the novice and vice versa.
Still, that is not enough to make search results truly relevant and personal. The personalization engine needs much more granular information about what the employee knows, how they prefer to learn, what is easy, and what is difficult for them, what type of information suits them best, and so on.
3) The third, much more advanced, step is to combine the approaches already described with the collection of information about all learning activities happening in real-time.
We are talking about using the Experience API (xAPI) in combination with the Learning Record Store (LRS). With xAPI it is possible to collect information about the employee’s learning happening in many places and with a great degree of detail.
- If the company intranet is xAPI enabled, it is possible to collect information about opened pages and downloaded documents.
- If the employee’s actions in a factory are tracked with some sensors, those could be collected as xAPI statements.
- If a company uses simulations or Virtual/Augmented Reality (VR/AR) for training, actions, and events inside the simulation could be tracked and stored as xAPI statements.
- If a company uses a Learning Experience Platform, like Valamis, for delivery of its training, all the user’s actions are automatically tracked with xAPI.
How to make learning even more personalized and effective?
When all that information about the employee is available to the personalization engine, it could make much more justified guesses about the relevance of some information for the employee.
By analyzing the learning history, it could become clear what learning format is most suitable for the person. For example, does reading an article contribute more to the learning than audio, do they prefer longer learning sessions with broad context presented or should it be short and directly to the subject, or do learning needs vary depending on the time of day or day of the week?
Even more relevant results could be produced when the employee’s knowledge and experience are compared to other employees’ experiences and similarities found in roles, skills, or learning activities. Then the relevance of the information provided to the employee could be improved based on that similarity.
This also works well for a new employee who has no previous history in a company. At first, similarities like role and department as well as an absence of previous learning history could bring them relevant onboarding materials, and then by analyzing the history of the previous newcomers, a recommendation engine will be feeding the learner with suggestions that are already proven to be relevant to those who came and went through onboarding materials before.
Needless to say, it is not the end of the story, it is only just the beginning. To bring it to the next level, the loop must be closed and learning should be applied to the personalization engine to produce better relevance over time. Analyzing learning activities, checking what employees were choosing themselves from results suggested, asking if they were satisfied with results provided, looking into refined searches – all that will make the recommendation engine improve and adapt all the time using machine learning.
How modern technologies can help with personalized learning?
There are many tools available, with more being developed every year, that are helping refine and redefine what personalized learning is.
Recommendations
Much like the ‘recommended for you’ section of Netflix is powered by an algorithm that looks at what you have enjoyed previously and delivers new content based on that information, recommendations can be used to seamlessly queue up learning content that is tailored to the individual learner’s needs and role, and helps identify and prevent skill gaps.
AI assistant
Using an AI assistant frees up instructors to serve the immediate learning needs of each individual by taking over the curation and recommendation of material.
An AI assistant knows the learner’s data, including what their skills are, the learning path that they are following, and delivers curated suggestions as to what the learner should engage with next.
Flexible learning paths
Each person learns differently and has a different level of base knowledge about a subject. If a company uses a strict learning path, there is a chance that information already known is repeated, the information needed is not delivered, and the learner will disengage.
By using a flexible learning path, the learner is offered information adapted specifically to them by technology.
Information already known is automatically excluded or touched on in a cursory manner as a revision, the relevant information is delivered in a fashion tailored to the learner, and advanced content is offered to high-level learners.
Natural Language Processing (NLP)
Natural language processing can be used to make searches for information better, faster, and more precise.
By accessing the power of NLP, learners can search for the exact information they need, whether in text or video and find exactly the answers they need to any question that they might have.
How to create a personalized learning plan?
To properly harness the power of this type of information delivery, a personalized learning plan should be implemented.
Personalized learning plan is a document that includes the short and long-term goals of the learner, covers their strengths, weaknesses, skills, and knowledge gaps, and sets out the learning plan that is best for that particular learner. It is the roadmap that the learner will follow to reach their educational or training goals.
1. Assess
A personalized learning plan always begins with an assessment. To know where you want to go, you must know where you are starting from!
Assessments can be made for specific roles or at a team or departmental level and can be used for several employees at the same time.
They should assess what information should be known, at what level, and should be able to identify specific knowledge gaps.
Once the learner has completed their assessment, this data can be input into the system, and the development of a learning plan can begin.
The same is true on a departmental and company-wide level. Data from teams can highlight the areas of weakness in a department, and show where their strengths are.
2. Determine the goals and skills needed for each specific role
Now that you know where you are starting from, you must next decide where you are heading.
It should be determined which skills or competencies are needed for each role or department.
By doing so, an organization is able to create or modify learning materials to develop or improve those skills.
Goals and skills should be created according to the SMART method, so that the learner’s, or the departments, progress in reaching them can be more easily tracked.
3. Create a generic learning plan
You have gathered data on the current level of employee’s knowledge and skills, and identified what they need to learn.
The next step is developing the path to get them there. A learning plan should be developed.
This doesn’t need to be hyper-specific – a generic series of steps that take the learner from point A to point B will suffice, and data will be gathered as more learners use this path to help personalize it for future learners.
4. Map learning modalities
Working to understand how the learner interacts with learning materials, and which materials will work best for this particular person is an important aspect of personalized learning.
Do they prefer video content? Perhaps they are more comfortable working with text and mini-quizzes.
Each person will have preferences for how they engage with the material, and this should be discovered at this step.
As it is discovered what works best for each person, this information should be mapped.
It is also good, at this stage, to create learner profiles. This profile not only can show the path of the individual learner and their accomplishments, but it can also serve as a blueprint for other learners in similar roles.
Understanding how each learner has been successful can be valuable data for a training program.
5. Modify learning plans for individuals according to their specific preferences
By customizing the learning plan, using data about the learner’s specific knowledge level, preference of content, and many other factors, an organization can ensure that learners are engaged, gaining skills, and not wasting their time on unnecessary content.
6. Utilize assessments to track learning
Recurring check-ins through assessments should be implemented to ensure that the learning path is successful.
These assessments, both one-on-one and self, should then deliver data to be analyzed that will help give a window into the learner’s journey.
One-on-one assessments are important here, as they allow for feedback, problem-solving, goal restructuring, and many other positive actions that can help learners be supported in reaching their goals.
A personalized learning plan is driven by this data, and the more data that there is, the more responsive it can be.
7. Review and scale
Throughout the program, the organization should review the process, continually work to improve the overall learner experience and make sure that the process itself is optimized.
This is also where an organization can update or scale a program.
Conclusion
Making learning personalized has a great impact on learning outcomes. When new concepts are linked to a person’s previous experience, it results in better understanding, and learning becomes more effective.
In an organizational environment, the creation of personalized learning requires technology solutions to make it cost-effective and scalable.
Technology, like a combination of Experience API (xAPI) and Learning Record Store (LRS), enables the collection of an employee’s experiences on a very granular level in digital form.
This information could be then leveraged in building a personalized learning experience in future learning activities using a combination of sophisticated search and personalized engines.
Of course, none of the technology solutions is perfect, but by closing the feedback loop from a learner’s activities back to the solution, the quality of answers given to a learner will be constantly improving.
Having that, instead of “figure out yourself” type of learning, employees could enjoy and experience truly personalized learning.
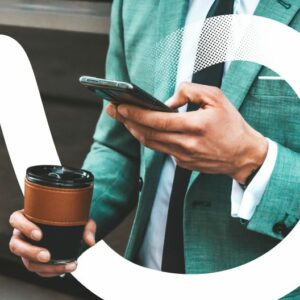
The definitive guide to microlearning
The what, why, and how-to guide to inject microlearning into your company.
DOWNLOAD GUIDE