What is a Learning Experience Platform?
Everything you need to know about the Learning Experience Platform: what is it, why LXPs emerged, key features & capabilities, LXP vs. LMS comparison.
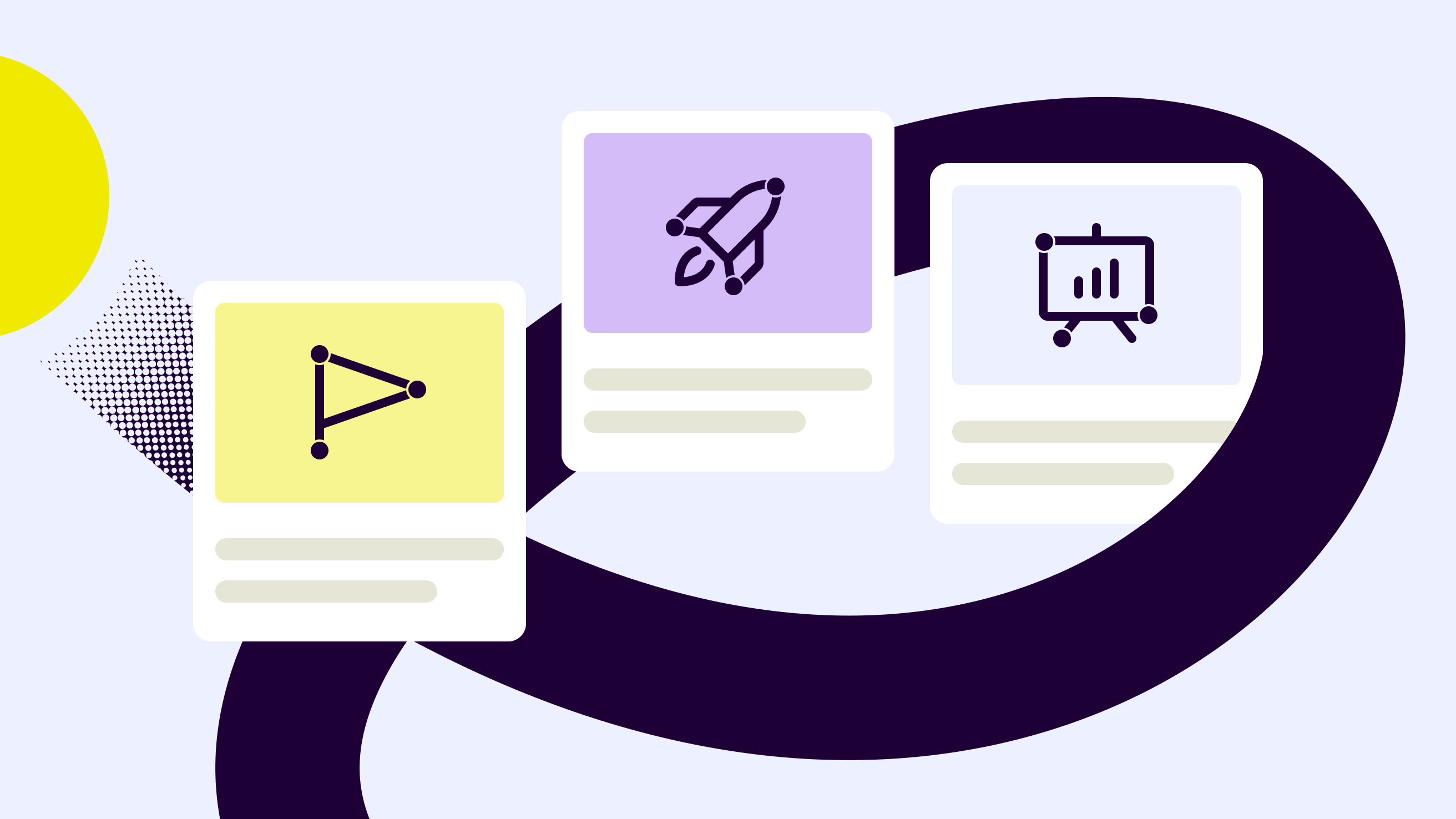
Organizations have been using old Learning Management Systems (LMSs) for a long time now. But as training requirements started changing, company executives, learning professionals and learners realized that there was something missing. There was a strong realization of the need for a more diverse and robust learning environment.
Discover:
- What is a Learning Experience Platform?
- The emergence of Learning Experience Platforms
- Key features of Learning Experience Platforms
- How do LXPs differ from LMSs?
- LXPs – Unlocking learner potential
Over the past half-decade or more, the corporate training landscape has seen a lot of innovation. More advanced approaches, such as lifelong learning, adaptive learning and engagement learning, are rapidly emerging to become mainstream.
Today, trainers deliver eLearning through a variety of specially designed tools and technologies that provide highly personalized learning.
The capabilities of those environments, combined with the unique types of experiences delivered to the learner, are what create Learning Experience Platforms (LXPs). And today, LXPs are a growing part of the learning ecosystem.
According to Josh Bersin, founder of Bersin by Deloitte: “…the LXP market itself is now over $350M in size and more than doubling every year. It’s also changing quickly”.
What is a Learning Experience Platform?
LXPs use digital technologies to integrate internal, external, and user-generated content, offering customizable learning experiences. They shift the approach to corporate learning by focusing on data and enabling more personalized interactions, making learning more engaging.
LXPs provide flexibility for L&D teams by integrating with existing systems, offering better support for both corporate and third-party tools. The openness of their architecture allows them to serve a wider audience and adapt to evolving technologies like AI and Data Analytics.
By analyzing data, LXPs can identify learning gaps and recommend strategies to address them, moving beyond traditional training to become the core of an organization’s learning infrastructure. This results in a more personalized, comprehensive learning experience for users.
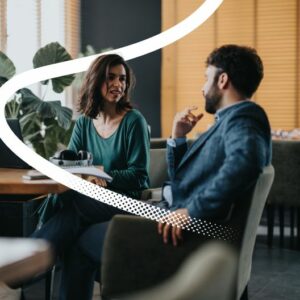
Checklist on how to choose the best LMS
The checklist will keep your focus on what really matters when choosing an LMS.
DownloadThe emergence of Learning Experience Platforms
Learning Experience Platforms emerged as a response to the limitations of traditional Learning Management Systems, which primarily deliver training content but fail to meet evolving enterprise training needs.
The shift toward experience-based platforms stemmed from the understanding that businesses must enhance their products and services to improve customer experiences.
Learner feedback on existing platforms, including LMS, highlighted the need for a more engaging and personalized learning experience, driving the development of LXPs.
1. A move towards personalization
The traditional LMS was designed around delivering content, but it didn’t prioritize the learner’s experience.
Users were often left sifting through a broad catalog of content, making it difficult to find relevant material.
Despite attempts to improve this with smart search features, the core issue remained: LMS platforms were designed more like a library, requiring users to already know what they needed before they could even begin their search.
In contrast, modern LXPs embrace a more user-centric approach.
By leveraging advanced technologies from major players like Google, Amazon, Facebook, and Apple, LXPs provide intuitive, personalized learning experiences.
They adapt to the learner’s preferences and needs, offering a streamlined, more engaging way to discover and consume content. This shift is driven by the fact that learners today are accustomed to personalized experiences in their personal lives and expect the same seamless interaction in their professional environments.
LXPs fill this gap, offering quick, effective learning that’s tailored to each individual, something LMSs failed to do.
2. Pushing the boundaries of standards
Another driver for the emergence of LXP’s is the standards adopted by modern-day LMS’s – which are SCORM-based.
While SCORM does “get results”, it is limited in what it can do. One of the main goals of any corporate learning platform is to connect learning with on-the-job performance. And SCORM makes it very difficult to decide how effective the courses really are, or how learners benefit from these courses.
Experience API (xAPI) on the other hand – the standard embraced by LXPs – offers significantly enhanced capabilities to the platform. When you use xAPI, you can follow different parameters both while you learn and perform on the job tasks. And, what’s even better is that you can do that on a variety of digital devices.
This allows you to track how learning influenced employee’s performance. For instance, with these new standards, you can now:
- Capture data from a vast array of sources
- Track learners activity via simulations and VR sessions
- Track where and how your employees learn (what devices they use)
- Assess to see how learners absorb the information in the course
- And most importantly, you can do all of this (and more!) using any device – so long as it can connect to the LXP either directly or remotely
All these advantages help you understand whether your training is truly effective and how it influences the work that people perform, which makes it easier to calculate ROI.
xAPI helps you understand what works and whether it makes sense to invest in something you wanted to invest originally and what should you really invest in.
3. Moving beyond “intelligence”
Many LMS solutions pride themselves as being “highly intelligent”. However, newer data processing and analytics technologies have pushed the need for enterprise learning solutions to go much beyond “intelligence”. And that’s exactly what LXP’s do!
Now, with the development of AI technologies everything has become even more interesting:
- Imagine you collect all this data about users, their behavior, performance, and learning, and provide it to an AI data engine. AI takes all that data and turns it into actionable information, telling you exactly what courses your learner needs to take.
- If you give it 1000 profiles, it will “learn” to predict what each learner needs based on patterns. This allows for preventing possible performance issues by recognizing them before they even occur.
But it doesn’t stop there!
Integrations are also possible with AI. If you integrate LXP and your Human Resource Management (HRM) system, the corporate intranet, your Learning Record Store (LRS) or the enterprise Customer Relationship Management (CRM) system, and collect the data from all of them, you can identify many different trends and patterns. And based on those patterns, all stakeholders can make informed training and learning decisions.
Standard LMS’s cannot do any of that. And though LMS developers are trying to get there, they’ve still got a long way to go to bridge the functionality gap with LXPs. As a result, there was an even greater impetus to the emergence of LXPs.
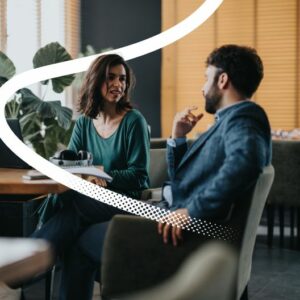
Is your company set for a digital learning solution?
You can quickly assess strengths, weaknesses, and areas for improvement with the template.
DOWNLOAD checklistKey features of Learning Experience Platforms
So, what should one expect to find in a typical LXP?
Well, one of the fundamental features of LXPs, that sets them apart from LMSs, is that they include sophisticated content discovery capabilities. Learners aren’t restricted by canned catalog content but can seek out interesting content for themselves, while also being guided towards relevant content by the platform.
Here are some other characteristics that set LXPs apart from LMS’s:
1. Extensive integration capabilities
Extensive integration capabilities that enable connection to a much-varied set of ecosystems through which learning can be furthered. For example, LXP-LRS integration can help deliver insightful information (trends and patterns) – through data analytics – that can aid in personalizing learning even further.
Integrating AI with LXPs can enhance learning experiences even further. Communication widgets and gadgets, like Learning Bots, can help customize unique learning paths for employees and serve as personalized learning assistants.
They remind learners about key learning milestones (courses due, upcoming assignment submission deadlines) and provide helpful revision and reinforcement tips. These tools also make training recommendations based on data analyzed from various sources, including corporate systems integrated with the platform.
2. Rich learning experience through deeper personalization
The ability to provide a richer learning experience through deeper personalization and more wide-spread learning opportunities. By integrating with other platforms – Google Services, YouTube, Social Media, Communicational tools e.g. communication leader Slack – LXPs extend the learning experience beyond the corporate learning system.
3. Supports various types of learning
Supports various types of learning, including problem-based learning, group-based learning, ILT, blended learning, through approaches such as Microlearning, Gamification and Adaptive Micro Learning.
4. Highly intuitive interfaces
They deliver content using highly intuitive interfaces, much like Netflix and Google do, based on past interactions and preferences. Through intelligent knowledge discovery (IKD), LXPs can provide highly adaptive, contextualized learning experiences based on the analysis of job performance, skills gaps and on-job competencies required.
Overall, when compared to LMSs, training delivered through LXP’s tend to be more:
- responsive,
- individualized,
- contextual,
- ubiquitous in delivery.
As a result, LXPs deliver highly immersive learning experiences to users of the platform.
How do LXPs differ from LMSs?
We defined that, typically LMS is a standalone learning platform used in a company to monitor, track, report on and administer employees’ learning activities. They usually serve as digitized online course catalogs. Like a library, learners go to the LMS portal to search for and complete a course.
For more details take a look at our blog: LXP vs. LMS: Which Platform to Choose in 2024?.
Following video helps to crystallize the fundamental difference between traditional learning management systems and modern learning experience platforms.
Summary
In summary, LMS’s help companies organize and manage employee learning needs. They do this by making learning content available to users, and then track and manage the consumption of that content.
In contrast, LXP’s act as knowledge enablers that deliver a more personalized learning experience to platform users. While LMS’s help employees uncover relevant content in its repositories, LXP’s enable them to discover it even further afield and through a much broader variety of sources, resources and mediums. And when integrated with data analytics engines, LXPs can offer powerful insights into key metrics, including ROI, business outcomes and links between learning and on-job performance.
LXPs – Unlocking learner potential
Advances in technology helped all stakeholders in the learning industry to realize that there are better and more effective ways to help learners unlock their potential and achieve their learning objectives. Technologies such as Artificial Intelligence, Virtual Assistants, Machine Learning and, Natural Language Processing have enabled the building of learning platforms that deliver far more enhanced levels of learner experiences than the previous generation LMSs could.
LXP’s can facilitate continuous learning across the company. They allow employees (learners) the flexibility to personalize what they learn, how they learn and when/where they choose to learn. They can identify specific skill gaps by understanding roles, analyzing performance, assessing career paths and proposing either remedial or progression learning.
LXPs are ideal for continuous learning. They can help employees enhance their productivity by fostering peer-learning or by making recommendations for mentors or “buddies”. They can also tap into social learning to ensure learning continues even after work.
A few years ago, because of corporate LMS platform limitations, the ability to integrate and download already-created courses or even purchase a membership/access to courses wasn’t even an option. Today, LXPs enable you to easily go to Coursera or any other external course provider and find a course you are looking for. And because of their powerful cross-platform support, you can even start taking your course on PC and finish it on your smartphone.
In search of better opportunities, employees today feel the need to continually improve their knowledge and skills – and not just for “statutory compliance”. Modern content services got people used to the idea that they can easily locate personalized and relevant content “on demand” – free from the corporate LMS network. HR managers also realized the benefits of being able to integrate critical processes like onboarding, performance measurement and learning management for the growth and development of their employees.
It was this intense need for newer, better, faster, measurable and more personalized experiences that have made enterprise LXPs a necessity.