Learning analytics
Understand what learning analytics is, and how to use it to improve learners’ outcomes and increase the effectiveness of your training programs.
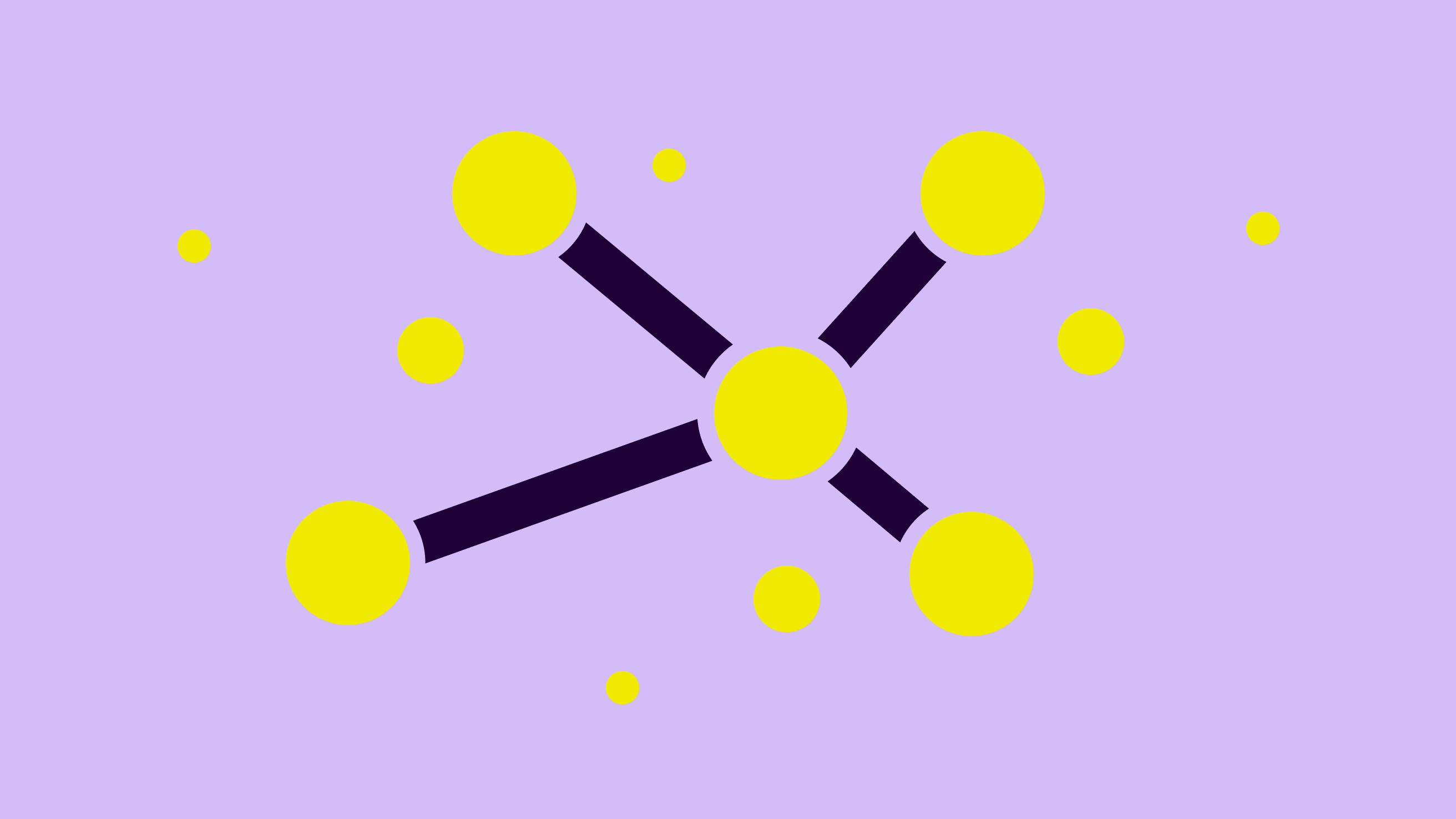
How do organizations understand the value produced by their training programs? They can rely on qualitative, anecdotal reports from supervisors and employees, or they can take a data-driven, analytical approach utilizing learning analytics.
In this guide, we will introduce you to learning analytics and how it can increase the efficacy of your training programs.
Discover:
- What is learning analytics?
- Why is learning analytics important?
- What are learning analytics methods?
- The challenges of implementing learning analytics
- How to implement learning analytics in your company
- Ethics and privacy in learning analytics
What is learning analytics?
Learning analytics is the collection and analysis of data to track learners and their interactions within an organization’s learning ecosystem.
The use of learning analytics can help understand the broader learning environment and uncover ways to improve overall learning outcomes.
Learning analytics examples – what data to track
Learning analytics can take many forms depending on how it is implemented. A good way to demonstrate what learning analytics can look like in practice is to consider the typical data it requires.
Learning analytics takes in employee data and outputs insights into their learning experience and current capabilities. To do this, organizations must track a range of employee data, including:
- Courses they have completed and passed, e.g., list of courses and any associated assessment scores
- Employee engagement in the training material, e.g., time spent on each course or post-training surveys they have provided
- Performance metrics showing their proficiency over time, e.g., their sales conversion rate, customer satisfaction rating, or other output/productivity metrics
- How they like to learn, e.g., the device they use, what time of the day they access educational material, their engagement level for various types of material
Why you need to use learning analytics
By tracking these kinds of information on your employees, you can develop and apply learning analytics in many ways. Examples include:
1. Measure key performance indicators (KPIs) before and after training
By tracking any changes in employee performance, you can assess the impact of training programs and pinpoint new areas where more help is needed.
2. Support learner development
Tracking data for learning analytics shows an organization what resources each employee needs to engage effectively with training material.
This saves time, helps employees improve in the most challenging areas, and shows employees the organization is actively involved in their development.
3. Understand and improve the effectiveness of training practices
Learning analytics shine a spotlight on the deficiencies in existing training programs.
Organizations can see each training course’s impact on the workforce, understanding what works, what doesn’t, and what needs updating.
4. Improve organizational agility
With a better understanding of the impact of training programs, organizations can adjust where needed, put money where it will be most helpful, and judge the overall direction an L&D initiative is headed.
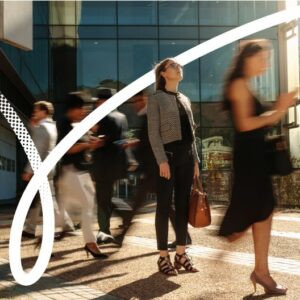
Use learning data to accelerate change
Understand learning data and receive a practical tool to help apply this knowledge in your company.
Download workbookWhy is learning analytics important?
By uncovering new patterns in the data, organizations can identify weak points in employees and their existing training practices.
When optimized, learning analytics can effectively find your best learning processes to maximize employee potential in the shortest time.
Through enhanced workforce capabilities, you can improve productivity and find new ways to innovate their operations.
These competitive advantages will only magnify the importance of learning analytics, and the investments organizations are willing to make in them.
1. Using learning analytics to enhance learning programs
There is a growing skills gap affecting the business world:
- A survey from Salesforce found 76% of employees don’t feel they have the skills they need to work in new digitally-focused workplaces.
- Deloitte found CEOs cited labor and skills shortages as the 2nd most crucial external factor hindering business strategies.
- 74% of hiring managers are struggling with a shortage of skilled candidates, according to The US Chamber of Commerce Foundation.
Employees and CEOs say there is a skills shortage, and hiring managers say recruitment can’t solve it. In that case, the only solution is to improve training programs and find ways to develop employees with the skills needed internally.
Learning analytics offers the detailed data needed to guide these future training programs. Without them, organizations cannot understand the efficacy of their L&D efforts. This includes:
- Identifying employees who aren’t currently served by existing training programs and need additional help
- Offering insights to help content creators build better learning paths
- Understanding the effectiveness of specific training programs
- Reducing L&D costs through more targeted training that generates better outcomes
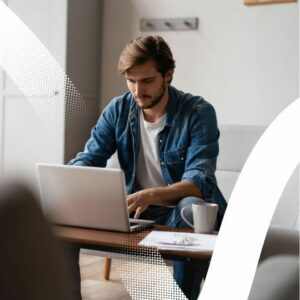
How to conduct a skills gap analysis and what to do next
Start building your foundation for strategic workforce development.
Download guide2. Using learning analytics to inform personalized learning experiences
Learning analytics can be applied on both the large scale, observing big-picture trends, and the small scale, discovering employee-specific insights. With this level of detail, organizations can deliver personalized learning experiences optimized for each employee.
This could be in terms of content (developing the skills most applicable to their career path) or delivery method (finding the proper form of learning for each employee). No two people learn in exactly the same way. By personalizing the learning experience, organizations can maximize the impact of training programs.
Learning analytics offers a range of tools to personalize the learning experience, including recommendation engines. These analyze employee data and learning history to recommend the most relevant content for each individual. Employees no longer have to search through educational material or follow orders from management or the L&D team. They can receive bespoke courses to fit their needs.
3. Generating employee engagement through learning analytics
Learning analytics deliver better training programs, and better training programs deliver engaged employees. A survey of US employees showed 92% found effective training improved their engagement. A LinkedIn report from 2022 showed learning opportunities were the most significant driver of workplace culture and employee engagement.
Engaged, knowledgeable employees have a better understanding of their work and how it fits into the broader organization. This helps increase productivity and employee satisfaction while also reducing turnover rates.
Read: 5 Ways to Improve Employee Engagement with L&D
4. The effect of learning analytics on employee retention
Most workers, 94% according to LinkedIn, are more likely to remain at a company if they invest in their L&D. Learning analytics helps produce learning support, personalization, and more. Showing employees they are valued and have a path to career progression if they choose to stay at the company.
This helps you hold onto and develop your best talent and build lasting, successful working relationships. Hiring is difficult and expensive. Gallup estimates the cost of replacing a single employee is 1.5 to 2x their annual salary. Avoiding employee turnover can make a significant impact on your bottom line.
5. Deploying L&D resources based on analytics
Learning analytics is perhaps an organization’s most powerful tool for understanding where to deploy its limited educational resources. In a perfect world, training budgets would be unlimited, as would the amount of time we could give learners to engage with it. But more and more, the time and budget available are shrinking.
Learning analytics helps pinpoint the most effective learning strategies, saving organizations time and money while delivering outstanding results.
6. How learning analytics can fix skills gaps
Many training programs aim to develop employee skill sets and fix skills gaps present in the workforce. With the introduction of new technologies and many roles becoming obsolete, organizations have to pivot to new ways of working, training their employees along the way.
However, building successful upskilling and reskilling programs is challenging. Research by McKinsey shows as little as one in three capability-building programs always or often achieve the desired results.
Successfully incorporating learning analytics into your training processes can help you get on the right side of that statistic. The advanced use of data means you can build a system to identify and correct skill gaps. Organizations can analyze the skill matrix for each employee in order to develop specific training programs or source new content to cover any gaps present.
Learning analytics methods
Within learning analytics, there are four main methods of analyzing the data collected from learners. Below are brief introductions to each method and links to more in-depth guides.
1. Descriptive analytics
Descriptive analytics is a statistical method used to search and summarize historical data in order to identify patterns or trends.
Data generated by interactions with the learning environment can be used to understand how they have previously behaved. This behavior can help inform how an organization’s current learning system is performing. However, descriptive analytics does not provide insights into a user’s future behavior.
2. Diagnostic analytics
If descriptive analytics tells you what happened in the past, diagnostic analytics tells you why it happened. It employs processes such as data mining, data discovery, drill down, and drill through, to highlight the causes of behaviors and events.
Diagnostic analytics is useful for identifying anomalies and helping organizations pinpoint areas that require further inquiry. It also uncovers causal relationships, showing how events might have resulted in the identified anomalies.
3. Predictive analytics
Predictive analytics is a statistical method that utilizes algorithms and machine learning to identify trends in data and predict future behaviors.
While descriptive and diagnostic analytics focus on the past, predictive is all about the future and identifying risks and opportunities. Using models such as decision trees, neural networks, and regression techniques, predictive analytics analyses past and present data to predict future trends.
4. Prescriptive analytics
Prescriptive analytics is a statistical method used to generate recommendations and make decisions based on the outcome of models. Considered an extension of predictive analytics, prescriptive analytics is not used as much due to the complexity of the machine learning it needs to function. However, it can be found within some learning experience platforms.
A platform using prescriptive analytics might be able to pinpoint areas of struggle for an employee, prompting the delivery of new content tailored to better suit the learner’s needs.
Janne Hietala, former Chief Visionary Officer at Valamis, explains how utilizing learning data can support learning and help drive better learning results.
The challenges of implementing learning analytics
As with any new technology, learning analytics brings unique problems that must be solved to get the most out of it.
1. Understanding the problem
First and foremost, you should understand the problem that you are seeking to solve with learning analytics. Without a purpose, even the most advanced learning analytic program will not be able to help you.
You cannot expect to implement learning analytics and magically solve all your problems. Instead, you need to start with a targeted approach and identify a specific problem you want to remedy.
2. Recognizing what must be built
As a relatively new field, learning analytics requires organizations to actively build their learning analytics programs.
Each company will have different digital environments and needs, each requiring a different solution. This is an area that can require significant trial and error, adjusting the program as it develops.
3. Understanding the target audience of your analytics
Many questions come up during the development of a new program:
- For whom are you developing this program?
- Will this be used solely for onboarding and training new employees? Or does it cover the entire employee lifecycle?
- Who will receive the data and take action?
- Will the company have dedicated roles, or will this be a task for departmental heads and managers?
These questions inform how the learning analytics program should be developed and managed.
4. Setting aside enough time to develop a proper program
While the first version of the learning analytics program will likely take a lot of time to implement, organizations should plan for multiple iterations.
As the program becomes active, faults and flaws will be uncovered that need adjusting. It’s not a one-and-done job.
5. Handling vast amounts of data
Data comes in a massive variety of formats, types, and locations.
Many organizations struggle to create a system that can analyze vast amounts of disparate information. Performance issues might be rampant, especially when the system scales to track more significant numbers of learners.
6. Creating programs that match expectations to technical capabilities
Learning analytics as a field has opened up many exciting doors. However, the field is still in its infancy, and there is currently much discussion about what it can do rather than what it actually does.
There might be an expectation that learning analytics will revolutionize your training program or completely change how customer behavior is understood. While both of these are possible, it is also true that there is a limit to what learning analytics can truly do.
7. Security
Finally, security is a massive challenge within this field. Handling this volume of data requires serious security considerations with regard to storage and access.
You should take steps to create an environment that ensures the safety and privacy of all who access it. This includes separating users’ rights according to roles and permissions compliant with EU GDPR and similar privacy laws.
How to implement learning analytics
1. Define your goals
As discussed in the previous section, you need a clear goal when implementing learning analytics into your training practices.
Without an understanding of the problem you are trying to solve, learning analytics can struggle to deliver the impact many hope for. This problem could be:
- Performing skill gap analysis
- Evaluating existing programs
- Improving L&D ROI
- Introducing personalized learning
- Specific operational benefits (e.g., improve site safety, increase conversion rates, etc.)
Or something completely different. Whatever you are trying to achieve, the first step to implementing learning analytics is defining your goal.
2. Tailor learning metrics
A key part of learning analytics is the specific metrics that track the learner experience.
There are many generic metrics that can be used to evaluate training programs (e.g., completion rates, employee performance metrics, etc.), but it is often worth going deeper and developing metrics specific to your aim.
For example, if you want to truly understand how learners interact with an existing course, you could track the following:
- The time it times to complete a course
- The relationship between the time spent and the final assessment score
- Social learning and how much discussion a course generates
- The time spent on each section or even a specific slide to see where employees are struggling
- Where employees lose interest or motivation by monitoring when they drop out or leave the learning platform for a break
With all the data available, you can use analytics to produce learning metrics specific to your needs.
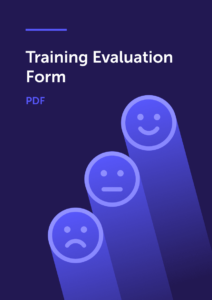
Training evaluation form
Get a handy printable form for evaluating training and course experiences.
Download now3. Learning analytics tools
Collecting all this data requires learning analytics tools, software that tracks, stores, and analyzes data, producing meaningful insights. While more traditional sources of learning data, such as surveys, still have value, they do not deliver the data required for learning analytics.
Common tools available to implement learning analytics include:
- Learning experience platform (LXP): software designed for building personalized learning experiences. LXPs come with a range of data collection and analysis capabilities ideal for the implementation of learning analytics. This includes a recommendation engine to find the most relevant educational material for each employee based on available data.
- Learning record store (LRS): software that stores learning records from various connected systems. LRSs are fundamental to the Experience API (xAPI) specification, a standard for tracking and sharing the learning experience across a number of platforms beyond the traditional learning management system (LMS). An LRS also offers features to follow learner progression, measure the effectiveness of educational content, and produce personalized programs. All critical parts of learning analytics implementation.
4. Data collection & storage
Whatever tools you end up using, the foundation of any learning analytics implementation is collecting extensive and accurate data. Ensure you have learning analytics tools integrated into every stage of your employee’s learning experience to generate as much data as possible about the training processes at your organization.
Once collected, ensure you have a safe and secure storage policy in place to protect user information. Discussed below are the ethical and privacy concerns inherent in learning analytics deployments.
If you are going to collect vast amounts of data and track your employee’s learning journey, you have to give them the courtesy of taking that responsibility seriously.
5. Analysis & action
The final step is the analysis and action. Learning from your data and putting the insights you derive into effect. This process involves data science processes, including:
- Clustering
- Relationship mining
- Discovery models
- Data disentanglement
- And the learning analytics methods discussed above
Undergoing these forms of analytics should deliver the insights needed to assist you in your L&D decisions moving forward. This could be the relative performance of various training material, the skills gaps most affecting your workforce, or information needed to improve the ROI from your L&D budget.
Ethics and privacy in learning analytics
When gathering data, organizations must be aware of any potential issues regarding its ethical use and storage and any additional privacy implications.
There are five general ethical areas that organizations need to consider when implementing learning analytics:
- Management, security, and the privacy of the stored data
- Data discovery based on the anonymized sources of information
- Logging of the information about data source access
- Accuracy and completeness of data
- Responsibility and obligation taking actions on knowledge derived from the data.
Organizations should have a plan for how each issue will be approached, what potential problems might be encountered, and what the most logical response to those problems would be.
Final thoughts
Learning analytics is a vital tool to ensure the success of a business’s L&D programs. Without the data to understand the employee learning experience, organizations are putting themselves at a disadvantage compared to their more advanced competitors.
Learning analytics offers the insight needed to ensure your organization gets the most out of its training processes, delivering a measurable impact while remaining cost-effective.
Additional resources
Listed below are additional resources if you want to learn more about learning analytics or some of the related topics that came up during the article.
- A Beginner’s Guide to Learning Analytics, – by Srinivasa K G & Muralidhar Kurni, April 19, 2021;
- Learning Analytics – Measurement Innovations to Support Employee Development, – by John R Mattox II, Mark Van Buren, & Jean Martin, September 3, 1016;
- Learning Analytics Explained, – By Niall Sclater, February 17, 2017;
- Rethink capabilities to emerge stronger from COVID-19, – Mckinsey, November 23, 2020.